VideoRAG: Our new product feature to revolutionize long-video analytics
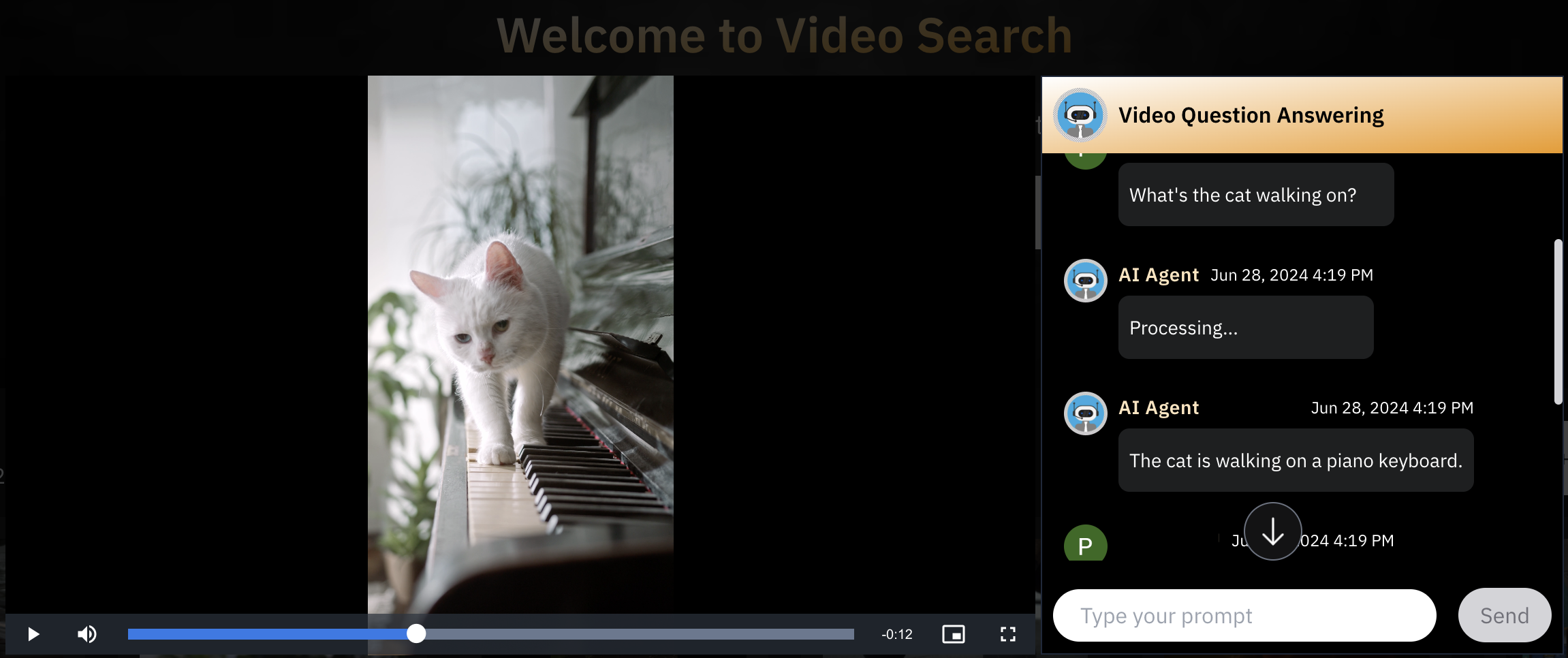
In the fast-evolving landscape of video analytics, finding precise information within lengthy videos has always been a significant challenge. As a significant enhancement to our recently launched product, Video Search, our innovative feature, Video Question-Answering (powered by VideoRAG) is poised to revolutionize the field. Unlike existing models and systems that struggle with the vastness and complexity of video data, VideoRAG leverages advanced Retrieval Augmented Generation (RAG) techniques to extract and deliver pinpoint information efficiently. In our quantitative experiments, we found an improvement of up to 23% when compared to a question answering system without RAG. Using this summarized information from the video, we can perform the question answering which is expected to improve the response and factual accuracy.
Architecture
.png)
The Video Search platform, which encodes, indexes, and retrieves videos, serves as the essential backbone supporting VideoRAG. The key components of VideoRAG architecture are as follows:
- Video Encoding: The Video Encoding component extracts diverse information from videos, including visual, textual, and audio data, and represents it in a high-dimensional embedding space optimized for vector search. It also catalogs structured details such as activity start and end times, tags, and captions, which are crucial for filtering and ranking results during retrieval.
- Retrieval: The Retrieval component efficiently matches user queries with pre-indexed video data. Upon receiving a text query, it uses semantic search techniques to fetch the most relevant video chunks from the vector database. By focusing on specific segments, this component ensures quicker and more relevant results, enhancing the foundation for the generation phase.
- Generation: In the Generation phase, the system uses retrieved video chunks to generate detailed responses to user queries. Leveraging advanced video question-answering models built with multimodal large language models, this component integrates visual, textual, and audio cues to produce accurate and contextually rich answers. This efficient and precise approach sets a new standard in video analytics and question answering.
VideoRAG Evaluation: Proven Superior Performance
To ensure the efficacy of our VideoRAG feature, we conducted a comprehensive evaluation using a dataset comprising long videos and 300 corresponding question-answer pairs. We assess three key metrics: Correctness of Information, ensuring generated text aligns accurately with video content; Detail Orientation, evaluating the completeness and specificity of the model’s responses; and Contextual Understanding, checking if the responses fit the overall context of the video. The metric values are scored using GPT-4, on the scale of 0–5, higher the better.
.png)
Here are a couple of examples of how VideoRAG can give you pinpoint and accurate answers based on your videos.


VideoRAG is able to pick the relevant information from the videos to answer the questions. Traditional systems lose on such minute details due to its architectural limitations.
Try our new feature!
VideoRAG is available on our Video Search platform. To experience this powerful feature, simply log in to the Video Search platform, select a video from your library, and open the chat window to start performing question-answering. It’s that easy! We invite you to explore VideoRAG and discover how effortlessly you can extract pinpoint information from your videos.